JSTOR invites you to explore an interactive research tool in beta. Developed in collaboration with our community, this tool employs AI and other technologies to empower people to deepen and expand their research with JSTOR’s trusted corpus.
Do you have questions, comments, or concerns? We want to hear from you! Please email support@jstor.org to share your feedback.
About the tool (beta)
The tool appears on the content page for journal articles, book chapters, and research reports, and as an addition to JSTOR’s standard keyword search. The tool helps you do the following:
Assess content relevance
The tool helps you more quickly assess whether a text is relevant to your research by highlighting key points and arguments, as well as explaining its connection to your search terms.
Deepen your research
Discover related topics, enrich your reading with similar content from the JSTOR corpus, and try new ways of searching.
Be conversational
Use natural, conversational language to ask questions and get quick answers about what you’re reading or researching.
The research tool in action
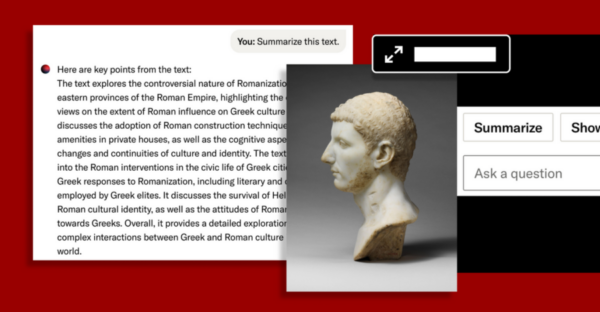
Explore how JSTOR’s interactive research tool empowers international students like Yuimi Hlasten by enhancing equity, efficiency, and accessibility in research.
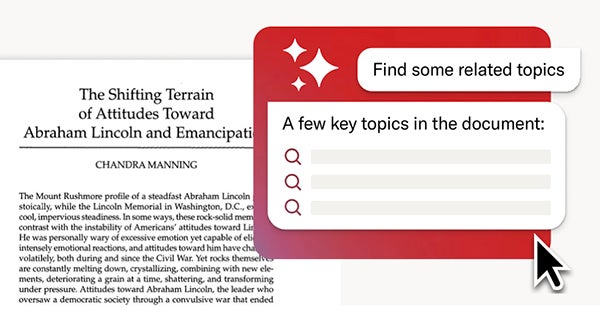
Discover how JSTOR’s interactive research tool helps educators like Bess Wilhelms and Steve Hermann streamline lesson planning, save time, and improve student engagement.
Frequently asked questions
- What does the tool do?
With the release of this tool, we aim to equip students, faculty, researchers, and librarians with innovative resources that facilitate engagement with complex content and enrich research and learning. The tool harnesses the power of AI to help users:
- Identify relevant material faster by surfacing key points and arguments from the text being viewed.
- Discover new topics and content within the JSTOR corpus, enabling exploration of additional possible paths of inquiry.
- Be conversational by asking questions about the text being viewed.
- Search JSTOR in a new way with a semantic search-powered capability that works better for natural language queries than traditional keyword search.
JSTOR stays up to date on the latest technologies and applies them in ways that best serve our users. JSTOR has previously applied machine learning (ML) and artificial intelligence (AI) technologies to optimize the research experience. For example, we have created a citation graph to link all articles on JSTOR, and used ML to improve the relevance of search results and recommendations. As we extend our knowledge and application of new technologies to AI, we expect to iterate and evolve as we learn. By using the tool and sharing your feedback, you will help us define the long-term scope of this exciting new feature.
- What content can the tool be used with?
At present, the tool can be used with journal articles, book chapters, and research reports found on JSTOR. Images, audio, video, and text-based primary sources on JSTOR are not yet included.
- What data sources is the tool drawing from to generate responses?
To start, we are using only the contents of the document being viewed to generate responses. Over time, as we learn from and improve upon the accuracy of responses, we might extend this to use the content of other relevant items within (but not beyond) the JSTOR corpus.
- Which large language models (LLMs) are JSTOR using?
The beta release of our interactive research tool uses GPT-4o-mini from OpenAI and the open source all-MiniLM-L6-v2 sentence transformer model. We are actively exploring alternatives and expect to evolve the models we use as our environment develops.
- How do you plan to measure or ensure accuracy?
We are monitoring and continuously improving accuracy using the following methods:
- Subject matter experts from a range of academic disciplines conduct in-depth, ongoing evaluations of the tool’s output. These evaluations help us ensure that generated content is useful and accurate.
- Users provide in-tool feedback that we use to identify areas for improvement. All interactions with the beta tool offer users the opportunity to provide a thumbs up or thumbs down rating. For thumbs down ratings, the user can provide further detail to explain their response.
- We assess model performance for our specific use cases using industry-standard metrics for Machine Learning (ML) and Natural Language Processing (NLP). Additionally, we continuously integrate new evaluation metrics specifically developed for Large Language Model (LLM) use cases.
- How will my information be kept, shared, and/or used?
JSTOR handles all personal information, including information provided to this tool, in accordance with our privacy policy. JSTOR does not sell user data, nor does it share content or user data from its platform for the purposes of training third-party large language models (LLMs). For more information about JSTOR’s privacy practices and the practices of the LLMs used by the tool, see “Data collection and use” in the Legal notices section of this page.
- Will there be fees associated with the tool?
Our goal is to offer the interactive research tool without additional fees. As a nonprofit, JSTOR’s financial model is designed to recover costs and support continued growth to meet the evolving needs of the educational community. We are therefore carefully evaluating the long-term costs of providing this service sustainably. By expanding early access, we are gathering critical usage data to finalize our cost model. This will allow us to make informed decisions about bringing these capabilities forward in an equitable and sustainable way.
- How is content protected from unauthorized or malicious use?
JSTOR maintains physical, technical, and administrative safeguards to protect the content we hold. We are a SOC2 compliant organization whose data security practices and measures are audited annually by independent third parties.
Content is only processed internally and with the OpenAI API. Please note that OpenAI API only temporarily stores data for processing purposes and does not use the submitted data to train their models or enhance their services. For more information on OpenAI’s data security practices, please consult the OpenAI Trust Portal and Amazon Bedrock policy.
- What is JSTOR’s overall approach to AI and other emerging technologies?
Technology has always been an incredible accelerator for ITHAKA’s mission to improve access to knowledge and education. As a trusted provider of scholarly materials, we have a responsibility to leverage content, technology, and deep subject matter expertise to chart a path forward that makes the use of AI safe, effective, and affordable for our constituents.
- We honor our values first and foremost. JSTOR provides users with a credible, scholarly research and learning experience. Our use of technology must enhance that credibility, not undermine it.
- We will listen closely and proceed cautiously. We recognize the concerns associated with AI and other emerging technologies, and are pursuing this work mindful of the very real considerations at hand. Our first step is to deepen our collective understanding through research and implementation in close collaboration with our community. We will use these tools safely and well.
- We empower people, we do not replace them. These tools should not be used to “do the work.” They should be designed to help people, especially students, deepen and expand their work.
- We will enable our systems to interact with users in ways that are comfortable. Traditionally, it has been the users’ responsibility to adapt to restricted language and structures to provide computers with inputs; computers can now interact effectively with users in natural language, and we should take advantage of that.
- We will lead with care. We will deeply consider the aspirations and trepidations of the many communities we serve.
As we learn about and pursue this latest technology, we look forward to your engagement and insights to ensure we continue to deliver high-quality, trusted, impactful services that improve access to knowledge and education for people everywhere. Learn more about how ITHAKA is exploring emerging technologies below.
- What does “beta” mean?
JSTOR’s interactive research tool is referred to as a “beta” feature because it is still in rapid development and in need of user feedback to help us develop it into the best tool it can be.
During the beta phase, we will work with engaged users to explore capabilities, foster innovation, refine functionality, and reveal limitations. As the tool evolves based on this work, its features may change. In this stage of development, the ability of the tool will be limited, and its functionality less stable than other tools on JSTOR.
- Will everyone get access to the tool eventually?
At this stage, we are gradually expanding access while gathering critical usage data to determine the best way to offer these capabilities equitably and sustainably. Although we aim to broaden availability, our priority is to ensure long-term accessibility within the educational community.
Promote the tool at your institution
Build awareness and engagement with JSTOR’s interactive research tool at your institution with these ready-made resources. From training materials and social media posts to customizable web copy, our toolkit has everything you need to help your community get the most out of this innovative tool.
Exploring new technologies at ITHAKA
ITHAKA offers a portfolio of nonprofit services, including JSTOR, Portico, and JSTOR Digital Stewardship Services, aligned around a shared mission to improve access to knowledge globally as affordably and sustainably as possible. Technology is central to achieving this goal. Through Ithaka S+R’s research and ongoing enhancements to JSTOR’s research and learning tools, we are actively exploring the use of AI and other emerging technologies to advance education and scholarship.
Approaching AI and advanced technologies together
JSTOR and ITHAKA’s founding president, Kevin Guthrie, shares his thoughts about our mission-driven approach to deploying new technologies to improve the learning and research experience for JSTOR users.
Generative AI and higher education
Ithaka S+R is working to uncover the implications of generative AI for higher education and discover its potential to support instructors, students, and researchers.
Sharing insights and learnings
We’re continuously sharing insights from real-world applications, usage, and user feedback. Stay updated on our latest findings on our blog.
Legal notices
Please keep the following in mind as you explore AI on JSTOR.
- Data collection and use
By using JSTOR’s interactive research tool, you consent to JSTOR collecting any data that you choose to share with the tool. JSTOR retains your conversation history in our logs and uses it in de-identified form, in accordance with our privacy policy, to maintain and improve the tool. We won’t ask you for any personal information, and request that you not share any in your conversations.
Any data sent to large language models (LLM) providers (which includes your prompt and some or all of the text of the content being viewed) is used only for generating the response. They do not use this to further train their models, nor do they retain the data for more than 30 days, in accordance with OpenAI’s API data usage policies.
- Beta limitations
This AI tool is currently in a beta state, therefore its features are subject to change without notice as we develop it. Current user experience may vary and may not be reflective of the tool’s future capabilities. Users should not rely on its outputs without conducting independent research. The tool should not be used to seek professional advice, including but not limited to legal, financial, and medical advice.
- Offensive materials and language
This evolving beta feature is built on JSTOR’s digital archive, which includes millions of items, spanning centuries and representing a wide range of ideas and perspectives. Given the historical nature of the materials on JSTOR, content items will reflect the era in which they were produced and/or the perspectives of the content creators, including the language, ideas, or other cultural standards of the time. Some content items may consist of or contain outdated language and ideas that are no longer in use and may be considered offensive, and the beta feature may repeat this when it summarizes or describes that content. Such responses do not reflect the views of JSTOR or its employees.
- Feedback
Any suggestions, ideas, or other information you would like to share regarding the tool may be used to improve, enhance, or develop its features. By submitting user feedback, you agree that such feedback becomes the sole property of JSTOR, and waive any rights, including intellectual property rights, related to the feedback.