Empowering research with JSTOR’s interactive research tool
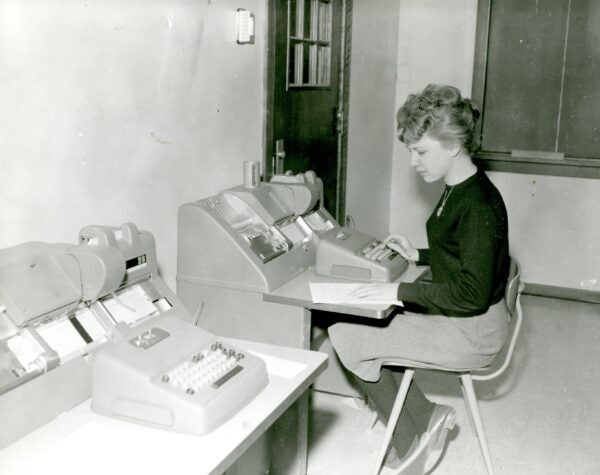
Fregley. Computer Center. 1964. Photograph, 4″ x 5″. Bucknell University; Lewisburg, Pennsylvania.
Since November 2022, ChatGPT has introduced new questions, worries, unknowns, and opportunities to the academic community JSTOR serves. Almost immediately, JSTOR began hearing directly from participants asking about our approach to machine learning and artificial intelligence in academia. While we don’t have all the answers, these conversations did open up an intriguing opportunity: to partner with our community to find solutions through collaborative research and practice.
While the collaborative work we’ve undertaken across JSTOR and our parent company, ITHAKA, has many facets, this blog post focuses on JSTOR’s interactive research tool, currently in limited beta. The tool helps users ask questions about articles, books, or research reports they are reading. It uses AI and other advanced technologies to solve common problems JSTOR users face: evaluating, finding, and understanding content.
All these problems impede JSTOR’s mission to expand knowledge access, insofar as “access” means the ability to not only retrieve information, but also to absorb and use it constructively. Early interviews reinforced our sense that traditional research necessitates time and skills that not all learners possess and are not necessarily required for comprehension of a given text. In what follows, I’ll unpack these obstacles, and explore how the beta tool is designed to address them while prioritizing credibility and transparency.
Content evaluation
Problem: Traditionally, researchers rely on metadata, abstracts, and the first few pages of an article to evaluate its relevance to their work. In humanities and social sciences scholarship, which makes up the majority of JSTOR’s content, many items lack abstracts, meaning scholars in these areas—who are our core cohort of users—have one less option for efficient evaluation.
When using a traditional keyword search in a scholarly database, a query might return thousands of articles that a user needs significant time and considerable skill to wade through, simply to ascertain which might in fact be relevant to what they’re looking for, before beginning their search in earnest.
Solution: We’ve introduced two capabilities to help make evaluation more efficient, with the aim of opening the researcher’s time for deeper reading and analysis:
- Summarize, which appears in the tool interface as “What is this text about,” provides users with concise descriptions of key document points. On the back-end, we’ve optimized the Large Language Model (LLM) prompt for a concise but thorough response, taking on the task of prompt engineering for the user by providing advanced direction to:
- Extract the background, purpose, and motivations of the text provided.
- Capture the intent of the author without drawing conclusions.
- Limit the response to a short paragraph to provide the most important ideas presented in the text.
- Search term context is automatically generated as soon as a user opens a text from search results, and provides information on how that text relates to the search terms the user has used. Whereas the summary allows the user to quickly assess what the item is about, this feature takes evaluation to the next level by automatically telling the user how the item is related to their search query, streamlining the evaluation process.
Discovering new paths for exploration
Problem: Once a researcher has discovered content of value to their work, it’s not always easy to know where to go from there. While JSTOR provides some resources, including a “Cited by” list as well as related texts and images, these pathways are limited in scope and not available for all texts. Especially for novice researchers, or those just getting started on a new project or exploring a novel area of literature, it can be needlessly difficult and frustrating to gain traction.
Solution: Two capabilities make further exploration less cumbersome, paving a smoother path for researchers to follow a line of inquiry:
- Recommended topics are designed to assist users, particularly those who may be less familiar with certain concepts, by helping them identify additional search terms or refine and narrow their existing searches. This feature generates a list of up to 10 potential related search queries based on the document’s content. Researchers can simply click to run these searches.
- Related content empowers users in two significant ways. First, it aids in quickly assessing the relevance of the current item by presenting a list of up to 10 conceptually similar items on JSTOR. This allows users to gauge the document’s helpfulness based on its relation to other relevant content. Second, this feature provides a pathway to more content, especially materials that may not have surfaced in the initial search. By generating a list of related items, complete with metadata and direct links, users can extend their research journey, uncovering additional sources that align with their interests and questions.
Supporting comprehension
Problem: You think you have found something that could be helpful for your work. It’s time to settle in and read the full document… work through the details, make sure they make sense, figure out how they fit into your thesis, etc. This all takes time and can be tedious, especially when working through many items.
Solution: To help ensure that users find high quality items, the tool incorporates a conversational element that allows users to query specific points of interest. This functionality, reminiscent of CTRL+F but for concepts, offers a quicker alternative to reading through lengthy documents.
By asking questions that can be answered by the text, users receive responses only if the information is present. The conversational interface adds an accessibility layer as well, making the tool more user-friendly and tailored to the diverse needs of the JSTOR user community.
Credibility and source transparency
We knew that, for our interactive research tool to truly address user problems, it would need to be held to extremely high standards of credibility and transparency. On the credibility side, JSTOR’s AI tool uses only the content of the item being viewed to generate answers to questions, effectively reducing hallucinations and misinformation.
On the transparency front, responses include inline references that highlight the specific snippet of text used, along with a link to the source page. This makes it clear to the user where the response came from (and that it is a credible source) and also helps them find the most relevant parts of the text.
Moving forward
Our August release of JSTOR’s interactive research tool marked the beginning of an ongoing cycle of learning and improvement. By incorporating feedback, analyzing behavior, conducting user research, and keeping pace with the rapidly changing technology, we are continuously enhancing the tool to meet the ever-evolving needs of the academic community.
On that front, we’re excited to begin extending the tool into the search experience, bringing the power of semantic search to the full JSTOR corpus of scholarly text. Stay tuned for more, and, if you haven’t already, sign up to try the beta tool to enhance your research experience and join our collaborative effort to harness this new technology in service of scholarship.
How to sign up for the beta
Users who are signed into a personal account and have institutional authentication on JSTOR will receive a pop-up that asks if they would like to sign up to try the tool. If you do not see this pop-up and believe you should, please email support@jstor.org.
Note that access to our testing environment will be limited so that we can create a controlled learning experience, where our product and technology teams can best study user interaction with these new features. We are not able to guarantee access to the tool. Learn more about JSTOR’s interactive research tool.